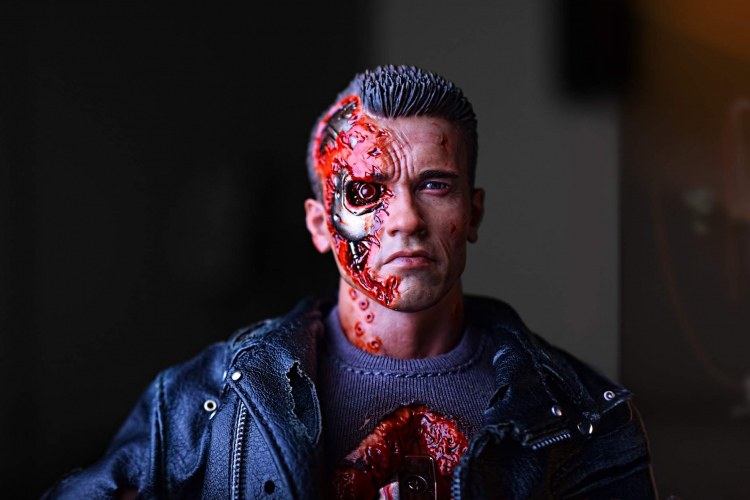
“The worst-case scenario for humanity with the development of super artificial intelligence is the potential for human extinction.” confesses GPT-4
In a previous article, we explored how decentralized cloud computing can compete with a powerful sector concentrated in Silicon Valley.
Using this power to fuel artificial superintelligence (ASI), AI surpassing human intelligence, will move us disturbingly close to our own extinction. Is this scenario really inescapable? Or can decentralized AI technologies avert the danger?
To understand what we are talking about, let’s set the stage. Please welcome Microsoft’s very own machine god.
What is centralized AI and how does it lead us to the machine god?
“AI isn’t separate. AI isn’t even in some senses, new. AI is us. It’s all of us.” Mustafa Suleyman, CEO of Microsoft AI, describes it as a new digital species in his Ted Talk “What Is an AI Anyway?”
“They communicate in our languages. They see what we see. They consume unimaginably large amounts of information. They have memory. They have personality.”
In a blog post titled Governance of superintelligence, authors Sam Altman, Greg Brockman, and Ilya Sutskever from OpenAI declare ASI to be inevitable – “So we have to get it right.”
“The prize for all of civilization is immense. We need solutions in health care and education, to our climate crisis.”
But what exactly is the cost to our civilization? Understanding the answer to this question will help grasp the significance of decentralized AI.
If god was Microsoft’s, would we be safe?
The central question remains – can we manage this new species, or will we give in to what Elon Musk estimates is a “10% or 20% chance” of human extinction?
Sam Altman, CEO of OpenAI, signed an open letter stating: “Mitigating the risk of extinction from AI should be a global priority alongside other societal-scale risks such as pandemics and nuclear war.”
To mitigate that risk, OpenAI suggests installing an oversight agency similar to the International Atomic Energy Agency (IAEA). This agency would set limits and track what fuels AI – computing power.
The machine god lives in a cloud-based supercomputer
Stargate is a $100 billion supercomputer set to launch in 2028 to train OpenAI’s superintelligence (ASI).
It’s symbolic of the continuation of a trend we’re seeing right now. The fuel of our next make-or-break technology is in the hands of a few superpowers: Amazon, Microsoft, and Google.
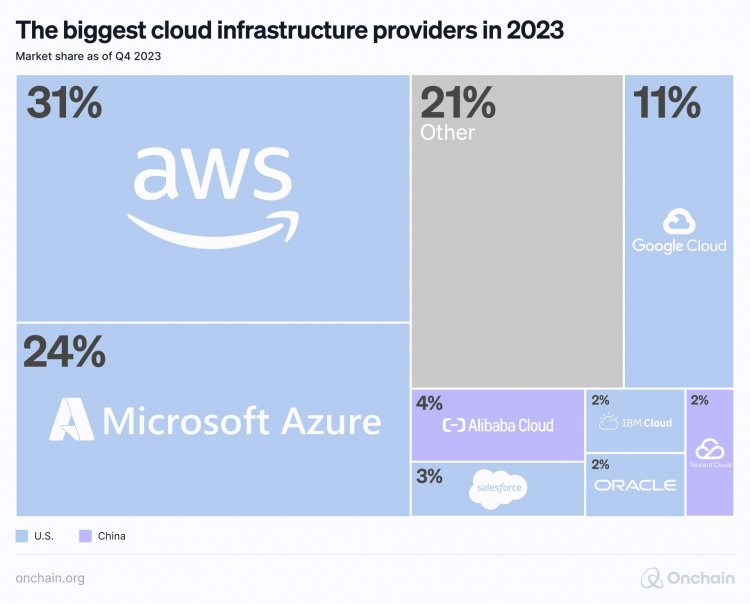
Since our extinction is on the table, let’s invest some human intelligence to ask if an oversight agency that can “inspect systems, require audits, test for compliance” is an appropriate response to this concentration of power.
You guessed the answer.
Shall we bring out our favorite hero?
Make blockchains, not power centers! Use decentralized computing power
Let’s add decentralized cloud computing to the equation.
On average, 85% of the world’s GPUs are idle, Hackernoon reports. Elsewhere, there’s a digital species on a diet. Can blockchain technology bridge this gap while breaking this concentration of power?
The Golem Network has released a roadmap to democratizing AI computing power. By installing Golem software, providers and consumers can exchange computing power. The software gives access to a decentralized pool that distributes the workload across the network’s GPUs.
Golem is definitely onto something. Zion Market Research predicts that GPU as a service will grow from $2.31 billion in 2022 to $28.7 billion in 2030.
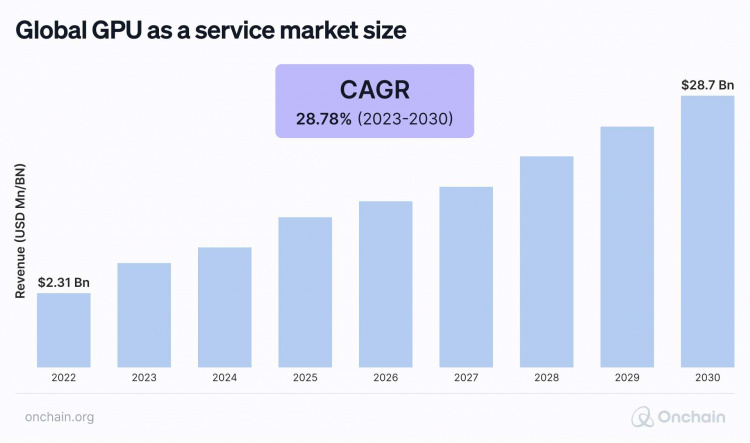
But in reality, blockchain won’t allow us to tap into the kind of computing needed to train LLMs (Large Language Models) and AGI (Artificial General Intelligence), the precursor to ASI that OpenAI is working on.
It’s simply a software/hardware mismatch.
Decentralized computing power won’t train centralized AI
Compute ≠ compute – and decentralized computing power won’t democratize AGI.
In fact, general-purpose GPUs promoted by Golem and Render Network, such as the Nvidia RTX 30xx series, won’t train LLMs. LLMs and AGI require specialized hardware.
State-of-the-art (SOTA) chips, like Nvidia’s A100 and H100 chips, are extremely effective when it comes to training LLMs. Training an LLM with trailing node AI GPUs (chips several generations behind the leading edge) would be at least 33 times more expensive than using enterprise SOTA chips.
Other decentralized computing networks like Akash offer these chips 85% cheaper than the big Silicon Valley cloud providers. But they are not immune to the SOTA GPU shortage.
Blockchain won’t fix the SOTA GPU shortage
As of 15 May 2024, the Akash Network has 71 SOTA (H100) chipsets available for its users.
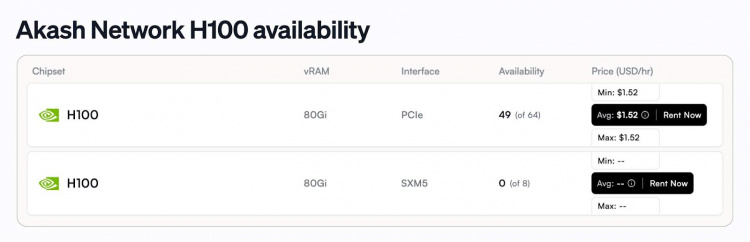
To put that into perspective:
- The supercomputer that trained GPT3 and is part of Microsoft’s Azure cloud computing platform contains more than 285,000 CPU cores and 10,000 GPUs.
- GPT4 was likely trained on 10,000 of Nvidia’s SOTA GPUs.
- Version 2 of xAI’s Grok LLM required 20,000 Nvidia H100 GPUs. Elon Musk anticipates that Version 3 will demand 100,000 H100 to train.
Stargate is likely to host even larger numbers of SOTA GPUs. The overwhelming demand for these chips further drives centralization within an industry already dominated by a few key players.

This problem is evident even within large corporations such as Alphabet, where the two competing AI teams, DeepMind and Google Brain, have had to merge due to limited data center capacity.
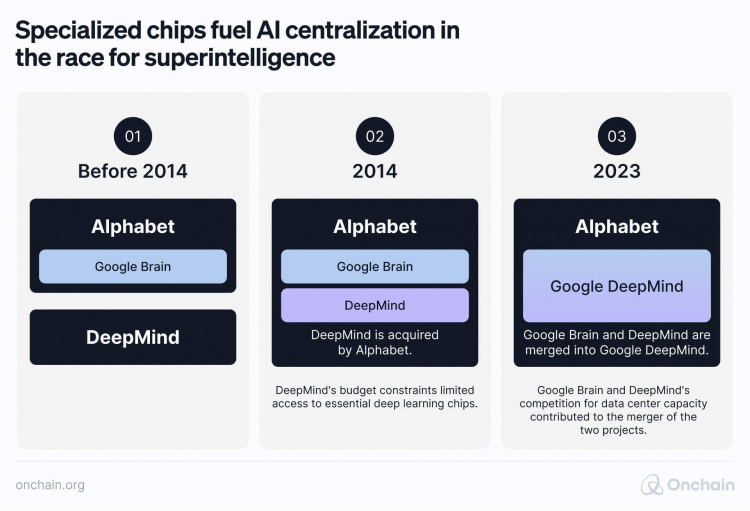
So is all hope lost? Perhaps not. Some ask why we should continue to develop ASI at all.
Why centralized AI might be a bad idea in the first place
On the Dangers of Stochastic Parrots is the name of a research paper published in 2021. It invites the reader to take a step back and ask, “Are ever-larger language models inevitable or necessary?”
Here’s what it says.
The Internet is a diverse space, but stochastic parrots are biased
The paper compares LLMs to parrots’ ability to absorb and respond to data. It expresses concerns about training LLMs and AGI on vast, uncurated Internet data. Data that carries biases from dominant social groups and underrepresents marginalized ones.
The environmental impact of centralized AI
The Stochastic Parrots paper goes on to stress the environmental impact of LLMs.
For instance, the Stargate supercomputer reportedly requires a five-gigawatt (GW) power source. A typical nuclear reactor provides one GW of power.
Big data and computing power are a substitute for poor data
Next, the authors argue that the pursuit of ever-larger models may divert research efforts from other valuable approaches that do not rely solely on increasing model size (and thus computing power).
The question is whether “the field of NLP (Natural Language Processing) or the public that it serves in fact need larger LMs?”
And “If not, what do we need instead?”
If any prominent figure in the AI field has an answer, it is Emad Mostaque, former CEO of Stability AI. “We didn’t figure out how to align humans – how to align AI, right?”
What is the difference between centralized and decentralized AI?
In March 2024, Emad Mostaque stepped down as CEO of the world’s leading open-source generative AI company, to pursue decentralized AI models.
Part of his decision stemmed from the fact that, as he argues, you can’t beat centralized AI with other, even bigger models.
For Emad it’s a question of “collective intelligence that is made up of amplified human intelligence…versus a collected intelligence and an AGI that is top-down and designed to effectively control us.”
What is decentralized artificial intelligence?
Emad argues that “big is a substitute for crap data”.
Swarm intelligence thrives on high-quality, localized data sets generated by globally coordinated teams in each sector and nation. This data then enhances sector-specific applications like education and healthcare.
It’s the result of multiple decentralized agents interacting and learning from each other. Because each agent trains on local data sets, swarm intelligence embodies the diversity found in human intelligence and enables adaptive problem-solving.
In contrast, centralized, top-down superintelligence represents a single point of failure and lacks the resilience of distributed systems.
Emad cautions about centralization risks, “A monolith is likely to be crazy…Geniuses are not mentally stable. Why would you expect an AGI to be so? You’re putting all your eggs in one basket versus creating a complex hierarchical system that is a hive mind.”
He advocates for a collective, decentralized form of AI—”the intelligence that represents us all”—as a safer and more effective alternative.
Blockchain technology enables this vision of decentralized AI models.
Blockchain for AI – the tech-stack for decentralized AI models
Ethereum plays a significant role in Emad Mostaque’s vision of decentralized AI. According to Emad, it provides the underlying, mature and accessible infrastructure needed for decentralized, secure and resilient operations.
This view is in line with the findings of our report, The Future Is Modular. While the report identified Ethereum’s layer 2 solutions as the superior blockchain architecture, it also outlines their challenges, including a high degree of centralization and limited scalability.
1. Data verification and attestation
Ethereum’s blockchain ensures the integrity and verifiability of data used by AI systems. This is essential for trust and reliability in decentralized environments where data comes from various sources and needs to be authenticated before use in training or decision-making processes.
2. Value transfer rails
Ethereum’s blockchain facilitates microtransactions between AI agents, which is critical because these agents need to exchange services or data. This capability allows AI systems to operate autonomously and interact economically within the ecosystem.
3. Decentralized governance
AI data governance must decentralize and democratize, removing control from a few dominant corporations.
4. Decentralized computing
While decentralized cloud computing will struggle to train centralized ASI, it will play a role in decentralized AI. It is, for example, already powerful enough to deploy Stability AI’s models on Render’s network of consumer GPUs. But it could also work in conjunction for decentralized AI training technologies like federated learning on the edge.
We are at a turning point in history – blockchain is the right direction
On September 26, 1983, Soviet duty officer Stanislav Petrov decided not to report an incoming U.S. missile attack that later turned out to be a false alarm, thus preventing a retaliatory nuclear strike.
We have been on the brink of disaster more than once. To risk it again is to ignore what history tells us.
Stanislav taught us courage, the lessons of the Cold War remind us to stop creating power centers. Understanding that we are interconnected is our evolution. It means building more and better networks.
Decentralized AI is emerging as a logical and ecological design.
Keep your eyes out for the upcoming Onchain report researching the synergy between AI and blockchain.